فهرست مطالب
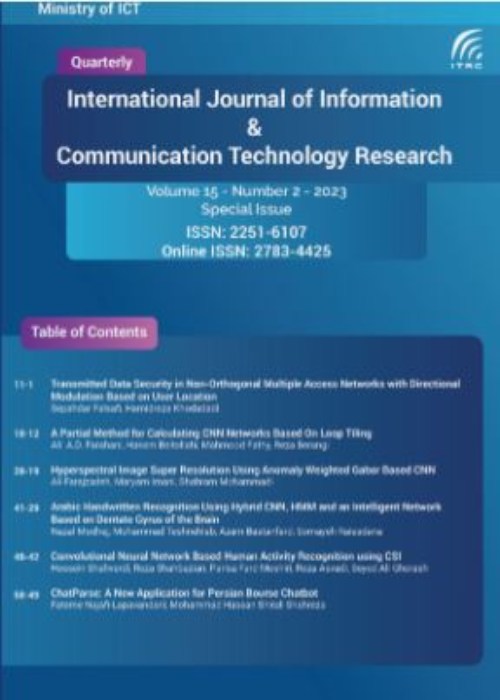
International Journal Information and Communication Technology Research
Volume:15 Issue: 2, Spring 2023
- تاریخ انتشار: 1402/04/24
- تعداد عناوین: 6
-
-
Pages 1-11
In Non-orthogonal multiple access, a user with a weaker channel gain is assigned more power than a user with a stronger channel. This type of power allocation allows the strong user (SU) to apply the successive interference cancellation (SIC) method that first detects the symbols of the weak user (WU) before detecting its data. The SIC method makes the WU data vulnerable to eavesdropping at the SU and so increases the detection complexity. This paper studies the physical layer security of the data against eavesdropping. The proposed scheme uses directional modulation that distorts the symbols transmitted in an undesired direction therefore the external eavesdroppers receive different symbols. It becomes complicated for an external eavesdropper to track and detect the signal of legitimate users. This scheme eliminates the WU data on the location of the SU so the strong user does not need the SIC. We analyze the impacts of various factors on security, such as the number of antennas and the secrecy rate.
Keywords: component, NOMA, directional modulation, DM-RFDA, eavesdropper, secrecy -
Pages 12-18
Convolutional Neural Networks (CNNs) have been widely deployed in the fields of artificial intelligence and computer vision. In these applications, the CNN part is the most computationally intensive. When these applications are run in an embedded device, the embedded processor can hardly handle the processing. This paper implements loop tiling to explain how one can construct a lightweight, low-power, and efficient CNN hardware accelerator for embedded computing devices. This method breaks a large CNN engine into small CNN engines and calculates them by low hardware resources. Finally, the results of small CNN engines are added and concatenated to construct the large CNN output. Using this method, a small accelerator can be configured to run a wide range of large CNNs. A small accelerator with one layer is designed to evaluate our methodology. Our initial investigations show that based on our methodology, the constructed accelerator can run a modified version of MobileNetV1, 70 times per second.
Keywords: Convolutional neural networks (CNNs), Hardware Accelerator, Embedded system, Low Power -
Pages 19-28
Hyperspectral images have high spectral resolution. But, due to the tradeoff between spectral and spatial resolution and various hardware constraints, imaging a hyperspectral image with high spatial resolution is not practical. Hyperspectral super resolution is a soft approach to solve this challenge. Recently, deep learning based methods such as convolutional neural network (CNN) show great success in this field. But, the contextual details in object boundaries and anomalies present in the scene are not well addressed. To this end, a new CNN based framework is proposed for hyperspectral image super resolution in this work. To improve ability of the convolutional blocks in simultaneous extraction of spectral and spatial characteristics, the weighted Gabor features are concatenated in output of the defined convolutional blocks. To extract more details containing anomalous targets present in the scene, the anomaly scores of pixels are calculated and used for weighting the Gabor features. The experiments on three real hyperspectral images acquired by AVIRIS and ROSIS sensors show superior performance of the proposed framework compared to several state-of-the-art methods based on CNN and residual networks. In addition to common super resolution metrics such as SAM and ERGAS, the efficiency of different methods are evaluated according to the classification accuracy metrics such as overall accuracy and kappa coefficient. The overall classification accuracy is increased from 70.39 to 88.23 in Indian dataset, from 86.07 to 96.20 in Pavia University dataset, and from 95.82 to 99.12 in Pavia center dataset.
Keywords: super resolution, hyperspectral image, anomaly, CNN -
Pages 29-41
Handwritten character recognition has occupied a substantial area due to its applications in several fields and is used widely in the modern world. Handwritten Arabic recognition is a major challenge because of the high similarity in its characters and its various writing styles. Deep learning algorithms have recently shown high performance in this area. The problem is that a deep learning algorithm requires large datasets for training. To overcome this problem, an efficient architecture is presented in this study, which comprises Hidden Markovian Model for character modeling, Convolutional Neural Network for feature extraction, and an intelligent network for recognition. The proposed network is modeled based on the dentate gyrus of the hippocampus of the brain. This part of the brain is responsible for identifying highly overlapping samples. The handwritten Arabic alphabet is characterized by this high overlap. Modeling the functionality of the dentate gyrus can improve the accuracy of the handwritten Arabic characters. Experiments are done using IFN/ENIT, CMATERdb3.3.1 and, MADBase datasets. The proposed approach outperformed recently published works using the same dataset. Although in all the experiments, a character error rate (CER) of less than 1.63 was obtained, the CMATERdb3.3.1 dataset resulted in a CER of 0.35. Compared with the convolutional neural network, the proposed network showed superiority in recognizing patterns with high noise.
Keywords: Handwritten Arabic recognition, Convolutional Neural Network, Hidden Markovian Model, Dentate Gyrus, Overlap -
Pages 42-48
Human activity recognition (HAR) has the potential to significantly impact applications such as health monitoring, context-aware systems, transportation, robotics, and smart cities. Because of the prevalence of wireless devices, the Wi-Fi-based approach has attracted a lot of attention among other existing methods such as sensor-based and vision-based HAR. Wi-Fi devices can be used to distinguish between daily activities such as "walking," "running," and "sleeping," which affect Wi-Fi signal propagation. This paper proposes a Deep Learning method for HAR tasks that makes use of channel state information (CSI). We convert the CSI data to RGB images and classify the activity recognition using a 2D-Convolutional Neural Network (CNN). We evaluate the performance of the proposed method on two publicly available datasets for CSI data. Our experiments show that converting data into RGB images improves performance and accuracy compared to our previous method by at least 5%.
Keywords: Activity Recognition, Channel State Information, Convolutional Neural Network, Deep Learning, WiFi -
Pages 49-58
In this paper, we design and develop a brand new application for Persian stock-market chatbot using the retrieval approach namely ChatParse. The proposed architecture for this system consists of the Persian version of the BERT called ParsBERT in which we also add fully-connected and softmax layers to consider the number of classes according to our designed dataset. We manually design an appropriate Persian dataset for bourse application including 17 classes because we have found no Persian corpus for this application. ChatParse is able to have multi-turn conversations with users on the stock-market topic. The performance of the proposed system is evaluated in terms of accuracy, recall, precision, and F1-score on validation set. We also examine our application with test data acquired from users in real time. The average accuracy of the validation set over 17 classes is 68.29% showing the effectiveness of ChatParse as a new Persian Chatbot.
Keywords: ChatParse, Persian Chatbot, stock-market, ParsBERT, attention.on